Mastering Mean Calculation for Financial Insights
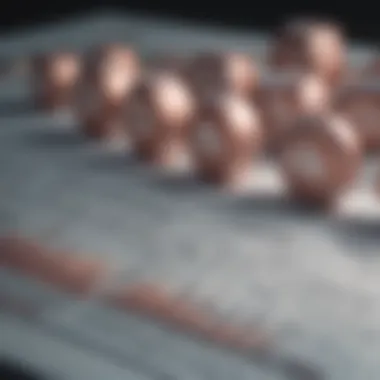
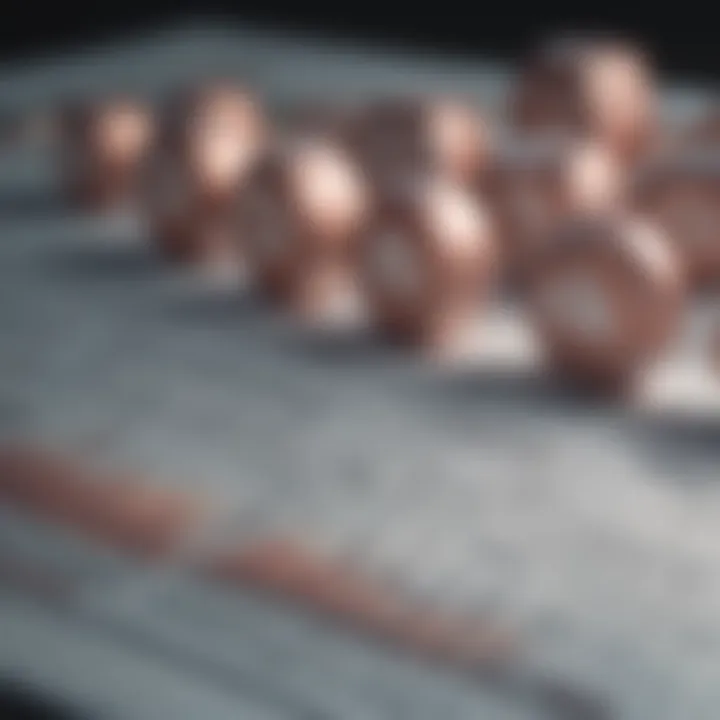
Intro
Calculating the mean is more than just a mathematical exercise; it serves as a crucial tool in finance and data analysis. By understanding this fundamental concept, readers can gain insights into market trends, make informed investment decisions, and enhance their analytical skills. This guide sets out to demystify the mean calculation, its application across various scenarios, and its relationships to other statistical measures such as the median and mode.
Through this narrative, we’ll explore how the mean is not merely a numerical value but a representation of central tendency, helping investors and analysts alike to grasp the essence of data trends.
"Statistics are like bikinis. What they reveal is suggestive, but what they conceal is vital.” — Aaron Levenstein
We’ll dive deep into the significance of the mean in making sense of financial data. Whether you're a seasoned investor, a financial advisor, or a student honing your analysis skills, distinguishing the mean will enhance your decision-making prowess. So, let’s break down the core elements that surround this vital concept.
Intro to the Mean
Understanding the mean is akin to grasping the lifeblood of data analysis; it's the heartbeat that often dictates important financial decisions and statistical interpretations. Whether you're evaluating the performance of a stock or assessing past sales figures, the mean provides a central point around which your data revolves, helping to simplify the hidden complexities.
Calculating the mean isn't merely a routine mathematical operation; it's a gateway to deeper insights into the behavior of data. Knowing how to compute the mean can assist finance students, analysts, and investors in grasping underlying trends or patterns that may not be immediately apparent. Think of the mean as a compass, directing your analytical journey through the often-turbulent waters of numbers.
Defining the Mean
At its core, the mean is a measure of central tendency that summarizes a set of values with a single number. Most commonly, it refers to the arithmetic mean, which is calculated by adding all numbers in a dataset and then dividing by the count of numbers.
For example, if you have a dataset representing daily sales: 200, 300, 250, and 350, the steps to find the mean would be:
- Sum up the values: 200 + 300 + 250 + 350 = 1100
- Count the entries: There are 4 values.
- Divide the total by the count: 1100 / 4 = 275.
Thus, the mean sales figure is 275. This simple yet effective calculation offers a snapshot of performance, allowing investors to quickly gauge overall success or areas needing attention.
Importance of the Mean in Statistics
The mean holds pivotal importance in the realm of statistics and data analysis for various reasons:
- Simplicity: The mean provides an uncomplicated snapshot of a dataset, making it an easy point of reference.
- Foundation for Analysis: Many statistical tests and data modelling techniques are premised on the mean; it's a cornerstone of inferential statistics.
- Comparative Benchmark: It serves as a benchmark against which other data points—especially outliers—can be assessed. This comparative approach is essential in financial analysis, as it allows for immediate recognition of deviations from expected performance.
"The mean acts as a guide, a statistical anchor that helps keep us grounded as we navigate through the sea of numbers."
In summary, while calculating the mean may seem like child's play, its significance extends far beyond simple arithmetic. It helps bridge the gap between raw numbers and actionable insights, transforming abstract data into a tangible narrative that shapes decisions and strategies in finance and beyond.
Calculating the Mean Step by Step
Calculating the mean is crucial in many fields, particularly in finance and statistics. Understanding this process allows individuals to make informed decisions based on their data. As such, knowing how to calculate the mean accurately can serve as a powerful tool in analysis and decision-making. This section will guide you through the fundamental steps involved in this procedure, clarifying the importance of diligence at each stage.
Collecting Data
Proper data collection is the bedrock upon which the entire calculation rests. If the data isn’t gathered meticulously or presents biases, the resulting mean may not represent an accurate picture of reality.
To gather data effectively, it’s important to define your parameters. What specific information do you need? For example, if you're looking at investment returns, collect data over a consistent time frame and ensure that you include all relevant investments. Inaccurate or incomplete data can skew the mean calculation, leading you astray.
- Identify your sample: What will you include in your data set? This decision needs careful thought.
- Choose a method: Whether it’s surveys, databases, or observation, the method should align with your objectives.
- Document your findings: Keeping a clear record of your data collection process ensures reliability and transparency later on.
Summing the Values
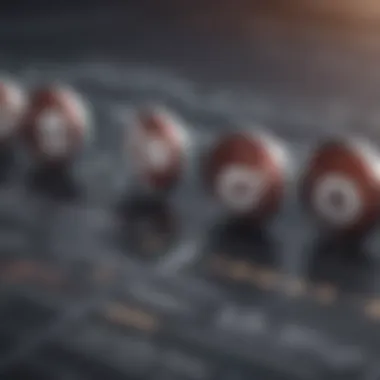
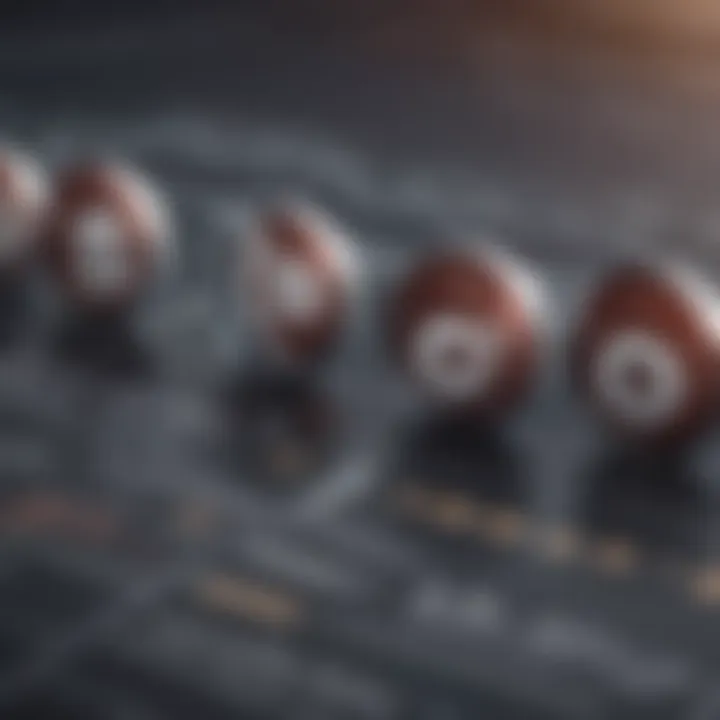
Once you've gathered your data, the next step is to sum the values. This essentially means adding everything together. While this step may seem straightforward, accuracy is key here. Adding mistakenly or omitting values often results in an incorrect mean, which can mislead decision-making down the line.
For instance, consider you collected returns from five different investments: $5,000, $7,500, $2,000, $3,500, and $4,000. The sum would be calculated as follows:
- 5,000 + 7,500 + 2,000 + 3,500 + 4,000 = 22,000
In such instances, it can be helpful to use a calculator or spreadsheet software to confirm the total. A small error in this step can have ripple effects on your final analysis.
Dividing by the Number of Values
After obtaining the total, the final step in calculating the mean involves dividing this sum by the number of values you have. This is where we derive the mean or average, allowing us to summarize the data comprehensively.
Continuing from the previous sum of $22,000, now divide this by the number of returns you collected, which is five in this case:
- 22,000 ÷ 5 = 4,400
Thus, the mean return for your data set is $4,400. Understanding each part of this calculation is important for grasping how averages work in your analyses. The mean can present an overall picture of the data, but care must be taken, especially when interpreting the value in the context of other statistics or external factors.
The mean is not just a number; it tells a story about the data.
By following these steps carefully, one can ensure reliable calculations that lead to sound financial judgments. In subsequent sections, we will explore different types of means to expand our understanding even further.
Types of Means
When discussing the concept of the mean, it's crucial to recognize that there isn't just one type that fits all scenarios. In fact, there are several types, each serving different purposes and yielding different insights. Understanding these distinctions helps not only in accurate data interpretation but also in effective decision-making, especially in fields like finance and analytics. When investors and analysts dive into financial data, they often lean on various means to derive significant conclusions. Here's a closer look at the primary types of means: the arithmetic mean, the geometric mean, and the harmonic mean.
Arithmetic Mean
The arithmetic mean is perhaps the most recognized form of the mean. It's calculated by summing all values in a data set and then dividing that total by the number of values. This method provides a straightforward average that is easy to compute and understand. For instance, if you were analyzing the yearly returns of a particular stock over five years, adding those returns together and dividing by five gives a clear picture of the average performance.
However, while the arithmetic mean is simple to grasp, it can be misleading when dealing with skewed data or outliers. If one year saw an exceptionally high return, it could distort the overall average, making it appear better than the reality for the remaining years. Thus, while the arithmetic mean is valuable for quick assessments, it’s essential to consider its limitations too.
Geometric Mean
The geometric mean, on the other hand, is often used for sets of numbers that are multiplied together, particularly in growth rates. Unlike the arithmetic mean, it involves taking the product of all values, followed by taking the nth root of that product (where n is the number of values).
To highlight its importance, consider an investor tracking returns across different years with varying performance rates. If one year features a return of 50% and the next a decline of 30%, the arithmetic mean will provide a misleading sense of stability. The geometric mean, however, will more accurately reflect the actual growth trend over those years.
A quick formula for calculating the geometric mean is as follows:
[ GM = (x_1 \times x_2 \times \times x_n)^1/n ]
Where x represents the data values. This formula shows that the geometric mean can provide insights that are especially valuable in inflation, investment growth, and other financial measures where multiplicative effects are at play.
Harmonic Mean
Lastly, we arrive at the harmonic mean, which is predominantly used in situations where rates are more relevant than absolute values. It's calculated by taking the reciprocal of the arithmetic mean of the reciprocals of the numbers. In simpler terms, you sum the reciprocals of the data points, and then take the reciprocal of that sum, ultimately providing a value that can be particularly beneficial in fields like finance when assessing average speeds or rates.
For instance, if an investor is examining the average price/earnings ratio of a collection of stocks, the harmonic mean would offer a more realistic viewpoint, especially since P/E ratios can vary significantly from one stock to another. The presence of outliers won't skew the data quite like it does with the arithmetic mean, making the harmonic mean a reliable tool in specific financial contexts.
"The right mean for the right situation will often yield clearer insights, making decision-making far easier in complex scenarios."
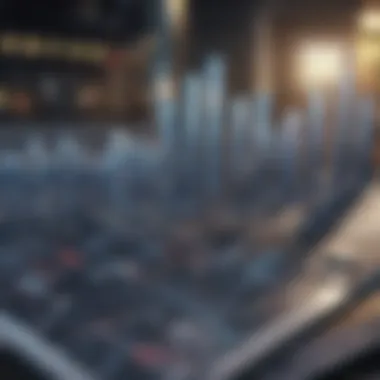
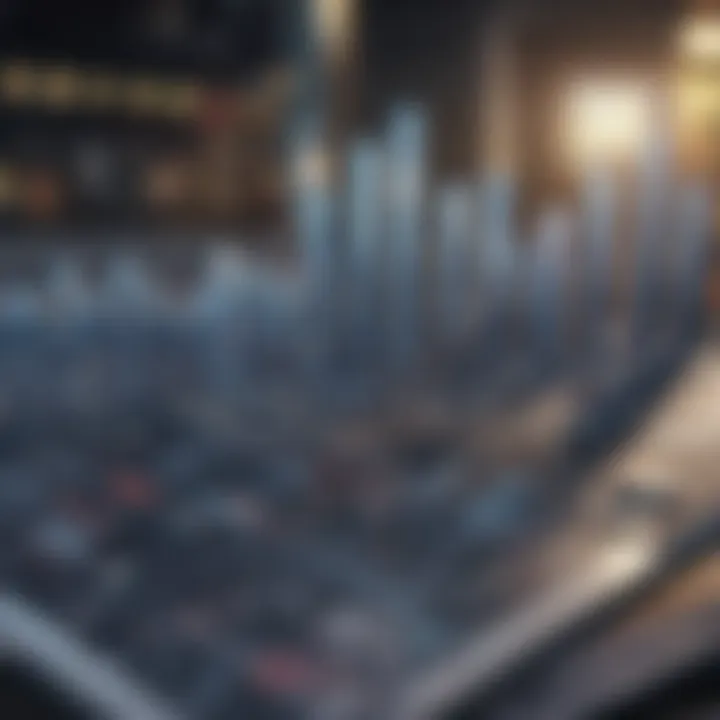
In summary, understanding the types of means and their respective applications can empower investors, analysts, and students to draw more accurate conclusions from data sets. It’s not just about finding the average—it's about knowing which average to use and why.
Mean vs. Other Measures of Central Tendency
Understanding how the mean interacts with other measures of central tendency is crucial for a well-rounded grasp of data analysis. The mean is often the go-to statistic for many, but it can sometimes tell only part of the story. In finance and other fields, depending solely on the mean may lead you astray, especially when other measures can provide deeper insights or a more accurate representation of the data.
Understanding the Median
The median is a robust measure of central tendency, especially useful in dealing with skewed distributions. Unlike the mean, which may be significantly affected by outliers—those rogue values that can skew the statistical results—the median simply finds the middle value of a sorted data set. For instance, if you have the following incomes: $25,000, $35,000, $45,000, and $1,000,000, the median income is $45,000. This gives a more believable picture of what an average member of that group earns.
The median's resilience to extreme values makes it an invaluable tool when evaluating things like income, home prices, or any dataset that might have a few high or low values that could pull the mean in a misleading direction. Using the median allows analysts to sidestep the influence of outliers, delivering a central point that perhaps showcases the data more accurately.
Exploring the Mode
The mode is another measure of central tendency that deserves attention, as it represents the most frequently occurring value in a dataset. It can be particularly useful when assessing categorical data where the average doesn’t hold much meaning. For example, consider a survey of participants' favorite ice cream flavors: chocolate, vanilla, chocolate, strawberry, and chocolate. The mode here is chocolate, highlighting its popularity among the survey group.
The mode can also be a useful statistic when you have data that comes in multiples, like age brackets in a demographic study. Since it highlights the most common value, it can point out trends or common characteristics that the mean and median might not emphasize. But like everything, it has its limitations, particularly in continuous data sets, where there may be no clear mode, or multiple modes exist (bimodal or multimodal).
Comparative Analysis of Central Tendency Measures
When weighing the mean, median, and mode, it's important to see what each brings to the table. The mean can be great when the data is symmetrically distributed and free of outliers. In those cases, every value contributes to the final calculation, so it's a true representation of the data.
Meanwhile, the median shines where outliers show up or where the data distribution is skewed. It provides the center in a way that remains insulated from those extreme values. And finally, the mode fills a unique niche, bringing forth the frequently repeated items that can show preference, trends, or behaviors.
Here are a few points to consider when choosing which measure to use:
- Data Skewness: If you know your data is skewed, prioritize the median.
- Outlier Sensitivity: Use the median for datasets with potential outlier values.
- Categorial Data: Utilize the mode for non-numeric sets to find common trends.
In many cases, a comprehensive analysis employs all three measures. Each has unique strengths and weaknesses, making them complementary. As an investor, analyst, or student, understanding their differences and applications can significantly impact your decision-making processes.
"Analyzing central tendency shouldn’t just focus on the mean; knowing how to work with the median and mode elevates your understanding and skills in data analysis."
Exploring these measures thoroughly prepares you to dive deeper into the complexities of your data, allowing for more informed and effective conclusions.
Applications of the Mean in Financial Analysis
The mean holds a significant role in financial analysis, acting as a pivotal metric that encompasses various aspects of investment evaluation, financial ratio assessments, and risk analysis. Understanding how to calculate and interpret the mean allows investors and analysts to make informed decisions based on financial data. It essentially serves as a foundational pillar for interpreting economic trends and forecasting potential outcomes. Within the realm of finance, applications of the mean enable stakeholders to discern patterns and guide investment strategies effectively.
Evaluating Investment Performance
Evaluating investment performance is one of the primary uses of the mean in financial contexts. By calculating the average return on investment (ROI) over a given period, investors gain a clearer perspective on how well their portfolios are performing. It’s not uncommon for an investor to look back over several months or years to determine what an average return looks like.
- Example: Suppose an investor tracks the quarterly returns of a mutual fund over four quarters: 5%, 7%, -1%, and 10%. By calculating the mean, the investor finds that the average return amounts to 5.25%. This information equips them with a benchmark for assessing whether to continue investing in that fund or explore other options.
Many investors often use comparisons against benchmarks, like the S&P 500 mean returns, to determine relative performance. The mean thus acts as an anchor, giving investors something concrete to reference against the volatility of returns.
Assessing Financial Ratios
Another crucial application of the mean is in the assessment of financial ratios. Ratios, such as the price-to-earnings (P/E) ratio, are commonly analyzed through their mean values over specific periods to identify trends in a company's performance.
- For instance: If a company, let's call it Tech Innovations Inc., had P/E ratios for the past five years as follows: 15, 18, 20, 17, and 19, the mean P/E ratio is 17.8. This average provides insight into how the market values Tech Innovations Inc. compared to its past valuations and against industry standards.
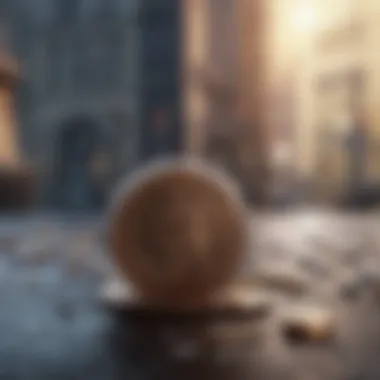
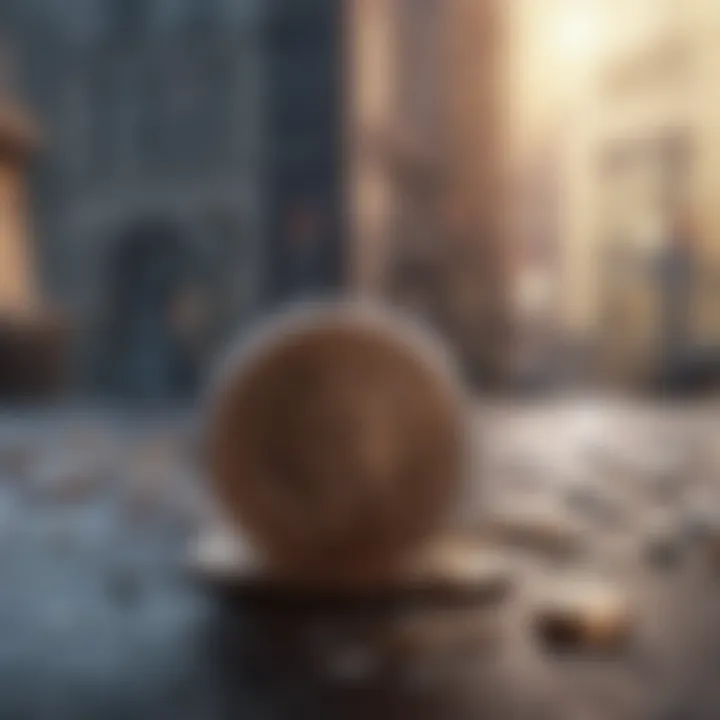
Understanding the mean of financial ratios can illuminate whether a company's performance is improving or degrading over time, providing essential levers for financial decision-making.
Risk Analysis and the Mean
Risk analysis is another area where the mean plays a fundamental role. The mean serves as a reference point for understanding expected returns and the volatility surrounding those returns, which is paramount in finance.
When analyzing risk, many investors calculate the standard deviation of returns, using the mean as a baseline. A larger deviation from the mean typically indicates higher risk. In simple terms:
- If an investment averages a return of 8% with a standard deviation of 2%, the expected range of returns would be between 6% and 10%.
- Conversely, if an investment sees high variability with a mean return of 5% and a standard deviation of 10%, investors could face returns that swing from -5% to 15%.
Understanding this relationship between the mean and risk helps investors make educated choices about which securities to include in their portfolios. As the saying goes, “don’t put all your eggs in one basket,” and comprehending how the mean plays into risk assessments underscores the wisdom in diversifying one's balance sheet.
Key Takeaway: Mastery of calculating the mean and understanding its implications can empower investors, financial advisors, and analysts alike, enabling them to identify opportunities and gaps in their analysis.
Common Errors in Calculating the Mean
Calculating the mean seems like a straightforward task at first glance, yet a multitude of errors can surface during this process. Miscalculating the mean can lead to misguided decisions, especially in finance and data analysis, where accuracy is pivotal. Understanding the common pitfalls is essential for anyone engaged in quantitative analysis, whether they’re statistical novices or experts. These errors not only compromise the integrity of data interpretation but can also lead to significant financial repercussions.
Misinterpretation of Data Sets
One of the most frequently encountered mistakes is the misinterpretation of the data sets used for mean calculations. Misunderstanding what the data represents or failing to recognize the context can result in skewed insights. For example, let’s consider a set of data points detailing the ages of a group of people. If we include everyone’s age, the mean will represent the overall age of the group. However, if we mistakenly exclude a significant portion, such as a batch of older individuals, the calculated mean could mislead one to think that the group is uniformly younger than it actually is.
Some key elements to consider:
- Sample Size: Larger, diverse samples typically yield a more reliable mean. Make sure the sample is representative.
- Data Categories: Recognize that categorical data behaves differently. For instance, averaging grades without consideration for grade distributions can mask poor performance in one area.
- Temporal Relevance: Data can become stale. As seasons or trends shift, past data quickly loses relevance, affecting mean calculations if inflated numbers are used.
Understanding the real meaning behind the data set informs the calculation and improves decision-making.
Impact of Outliers on the Mean
Outliers, those aberrant data points that seem out of place, can significantly distort the calculated mean. They can either inflate or deflate the mean in ways that do not accurately reflect the rest of the data set. For instance, if a financial analyst is examining salaries within a company and one executive earns a salary much higher than all other employees, the calculated mean salary could suggest a misleadingly high average.
Considering the effect of outliers:
- Identification: Before calculating the mean, identify any potential outliers using methods like the interquartile range (IQR).
- Adjustment: Sometimes it’s appropriate to exclude outliers from mean calculations to achieve a more accurate picture, but this must be done judiciously.
- Alternative Measures: When outliers heavily influence the mean, think about using the median or mode as a more stable measure of central tendency.
As a general rule, don’t let a few numbers throw you off course. Always question whether an observed mean adequately represents the underlying data.
Culmination
Understanding the mean is not just a mathematical exercise; it plays a crucial role in our daily lives, particularly in fields like finance and economics. The mean serves as a core measure that helps us simplify complex data into a single representative figure. This article has explored the calculation of the mean, its significance, and the common pitfalls encountered along the way. By breaking down the steps for calculating the mean and illustrating its relevance with practical examples, we've highlighted its importance as a foundational statistic.
Recap of Key Concepts
As we wind down, it’s important to revisit some key takeaways:
- Definition: The mean is the sum of all values divided by the number of values. It is the average that succinctly summarizes a dataset.
- Types of Mean: Apart from the arithmetic mean, we examined the geometric and harmonic means, all offering different perspectives depending on the context.
- Comparison with Other Measures: We delved into how the mean compares with the median and mode, emphasizing when one might be more appropriate than the others.
- Applications in Finance: The mean aids in gauging investment performance and financial ratios, making it indispensable for investors and financial analysts.
- Common Errors: We discussed issues like misinterpretation of data and the influence of outliers, showcasing the necessity of careful data analysis.
"The mean is like a compass in the vast sea of data; it guides us to the central point of understanding."
Final Thoughts on the Mean
As we conclude, it's vital to stress that while the mean offers valuable insights, one must approach it with caution. A dataset can have various characteristics, and thus, relying solely on the mean can sometimes lead to misguided interpretations. Especially in financial analysis, where stakes are high, ensuring accuracy and understanding of the context is paramount.
Looking ahead, it’s advisable for analysts and decision-makers to complement the mean with other descriptive measures and intuition about the data. Employing a combination of approaches can yield a more nuanced view, ultimately leading to better decision-making.
In summary, grasping the mean and its implications enriches not only our analytical toolkit but also enhances our comprehension of data, empowering us to make more informed choices.