Harnessing AI for Innovative Stock Trading Strategies
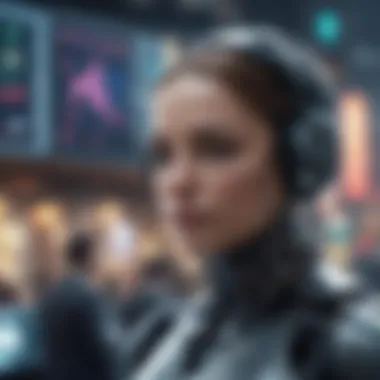
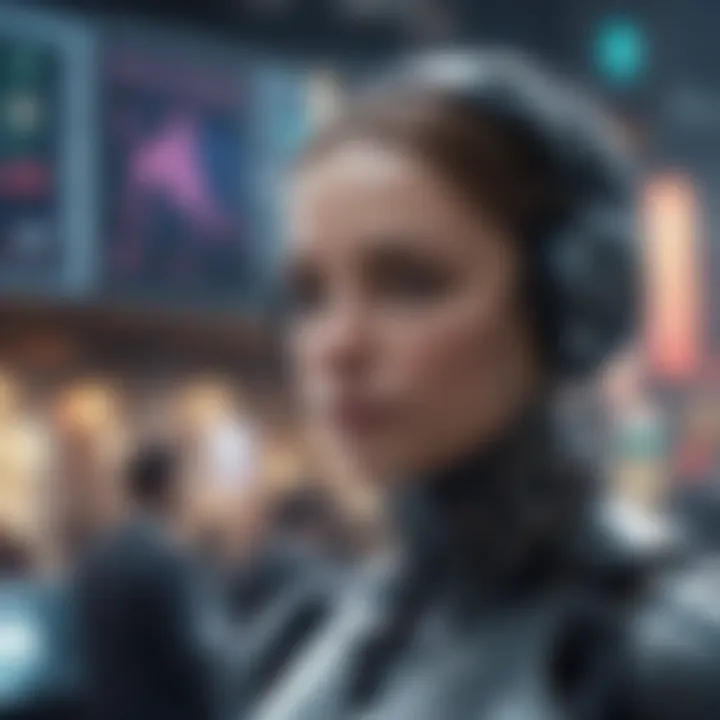
Intro
In the complex world of stock trading, adapting to changes is not just beneficial; it's crucial. The rise of artificial intelligence (AI) is restructuring this landscape in a profound way. AI is not merely a buzzword; it's a game changer. As traders grapple with swift market movements and vast amounts of data, AI's capabilities provide an edge that humans often struggle to achieve alone. But what does this mean for both seasoned investors and novices alike?
Understanding the underlying methodologies of AI in stock trading can unveil several distinct advantages over traditional strategies. From algorithmic trading to predictive analytics, the implementation of AI transforms decision-making processes and enhances the efficiency of trading systems. Moreover, as we delve into its implications, we will spotlight how this newfound technology is not just for elite traders; it holds the potential to empower investors across the spectrum.
This exploration into AI-driven trading systems aims to equip you with insights that go beyond surface level. As we dissect the components of AI in stock trading, we will affirm its relevance in today's financial markets and sketch the contours of what lies ahead.
Investment Dictionary
Navigating the depths of stock trading, especially when enriched by AI, requires a firm grasp of relevant terminology. Here are key terms that will illuminate the discussion that follows.
Key Terminology Breakdown
- Algorithmic Trading: This relies on automated, pre-programmed trading instructions to execute trades at optimal times and prices based on various conditions.
- Machine Learning (ML): A subset of AI where algorithms improve through experience, honing their predictive capabilities by analyzing past data.
- Sentiment Analysis: This technique analyzes text data from news, social media, and forums to gauge public sentiment regarding investments, influencing trading decisions.
- High-frequency Trading (HFT): A specialized form of algorithmic trading that involves executing numerous orders at extremely high speeds.
- Portfolio Optimization: This involves utilizing AI algorithms to adjust asset distribution within a portfolio to maximize returns and minimize risk.
Common Investment Types Explained
Understanding investment types is vital when integrating AI into trading strategies. Here’s a breakdown:
- Stocks: Ownership shares in a company. AI can analyze market trends and company performance to guide stock selection.
- Bonds: Loans to corporations or governments, offering fixed interest over time. AI can optimize the timing for buying or selling bonds based on market forecasts.
- Exchange-Traded Funds (ETFs): These track various indexes and can be traded like stocks. Machine learning can aid in selecting optimal ETFs based on market conditions.
- Options: Contracts that give the buyer the right, but not the obligation, to buy or sell an underlying asset at a set price within a specific time frame. AI can assist in the analysis of options trading strategies by processing complex data sets rapidly.
"AI in stock trading is not just about speed—it's about intelligence. The capacity to analyze patterns that the human eye might miss can lead to significant insights and opportunities."
Grasping these terms sets a foundation for understanding the later sections, which will discuss the methodologies and advantages of AI in stock trading.
Preface to AI in Stock Trading
Artificial Intelligence has shifted the landscape of stock trading significantly over the past several years. The importance of understanding how AI can be leveraged in this arena cannot be overstated. As financial markets continue to evolve at breakneck speeds, it is imperative for investors, analysts, and even casual traders to adapt and harness the power of technology to maintain a competitive edge.
AI in stock trading helps in numerous ways, from analyzing vast datasets in mere seconds to improving predictive accuracy, thereby influencing decision-making processes like never before. Traditional methods, which relied heavily on human intuition and manual analysis, are now being supplemented, if not replaced, by algorithms that can assess trends that a human may miss.
Additionally, the predictive capabilities of AI can yield insights that benefit not just large institutional investors but also retail traders looking to optimize their trading strategies. As we delve deeper into this article, key concepts will come to light, showcasing how AI technologies align with contemporary market dynamics. Here are some crucial elements this discussion will cover:
- The significance of AI in analyzing market trends.
- The transformation of trading strategies through technological advancements.
- Potential risks and considerations when incorporating AI into trading decisions.
By the end, readers will be equipped with a clearer understanding of how to capitalize on AI for their trading endeavors, irrespective of their expertise level.
Understanding AI: A Brief Overview
Artificial Intelligence, in its essence, refers to systems designed to perform tasks that typically require human intelligence. This includes learning, reasoning, problem-solving, perception, and language understanding. When talking about AI in trading, we're mostly referring to various subsets of AI technology, such as machine learning and data analytics, that allow traders to sift through mountains of data to make informed decisions.
There are multiple types of AI technologies, including:
- Supervised learning: This involves training a model on historical data to make predictions about unseen data.
- Unsupervised learning: This type does not use labeled data, allowing the system to find patterns on its own.
- Reinforcement learning: Models learn by trial and error, receiving feedback from actions performed.
These diverse technologies pave the way for new trading methodologies that can analyze sentiment, execute trades at optimal moments, and identify patterns invisible to the naked eye.
The Evolution of Trading Strategies
Trading strategies have come a long way since the inception of the stock market. Initially, trading was a hands-on affair — buyers and sellers would meet face-to-face at stock exchanges. As technology advanced, so did trading methods. The introduction of electronic trading platforms marked the beginning of a new era.
With the arrival of AI, trading strategies became even more sophisticated. The modern algorithmic trading strategies leverage vast datasets and computational power to make trades in microseconds, a speed unimaginable in previous decades.
Key milestones in this evolution include:
- Algorithmic Trading: Spurred by technological advancements, traders began using algorithms to automate trading processes, focusing on speed and efficiency.
- High-Frequency Trading (HFT): This critical advancement allows traders to execute thousands of trades in the blink of an eye, targeting minuscule price changes.
- Quantitative Trading: Data-driven strategies emerged, utilizing complex mathematical models to forecast market behaviors.
As AI continues to develop, it shapes how traders analyze data, determine risks, and make decisions, enabling them to weather market fluctuations better than before. This change encapsulates a fundamental shift in how market participants approach trading and invest in financial instruments.
The Role of Data in AI Trading
In the world of stock trading, data acts as the lifeblood for any successful strategy. Without accurate and relevant data, any artificial intelligence system would resemble a ship adrift in uncharted waters. Understanding the role of data in AI trading not only sheds light on the techniques used in the industry but also emphasizes the critical factors that traders must consider when delving into this sphere.
AI-driven trading systems heavily depend on data. They leverage different types of information to make informed predictions about market trends and asset movements. Traders who master the art of data utilization hold a significant advantage in the fast-paced environment of stock exchanges.
Types of Data Utilized
When discussing the types of data used in AI trading, it’s crucial to know that a broad spectrum is at play. Here are some principal categories:
- Market Data: This includes real-time price movements, volume, and order books. Market data allows algorithms to detect patterns and fluctuations rapidly.
- Fundamental Data: Financial statements, earnings reports, and economic indicators fall under this category. Understanding a company's health through these metrics is vital for long-term investment strategies.
- Alternative Data: This has become a game-changer. It can involve satellite imagery, social media sentiment, and even news headlines. Investors are increasingly harnessing such unconventional sources of data to gain insights that traditional models might miss.
- Historical Data: Storing and analyzing past data can enhance prediction accuracy. AI systems utilize this data to recognize recurring trends and market cycles.
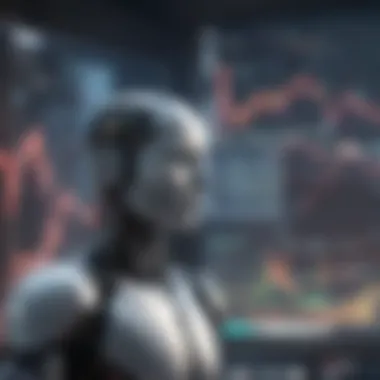
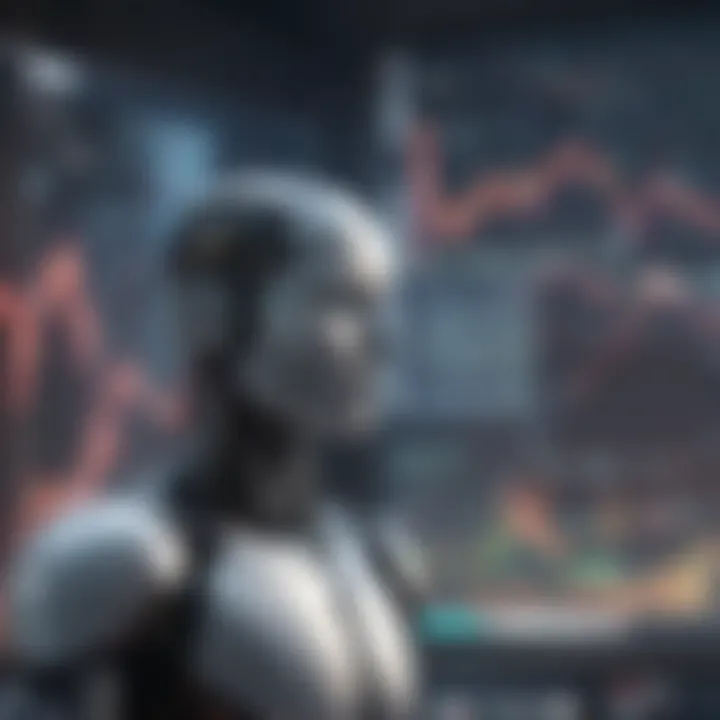
Data is not a one-size-fits-all scenario. Depending on a trader's focus—be it long-term investments or short-term gains—the types of data will greatly vary.
Data Sources: Where to Find Relevant Information
Tracking down reliable data sources is paramount for effective AI trading. Here are some common avenues traders can explore:
- Data Providers: Companies like Bloomberg or Refinitiv offer comprehensive datasets tailored for financial professionals. Their platforms provide various market data and analytical tools.
- Publicly Available Financial Statements: Accessing filings from the Securities and Exchange Commission (SEC) can reveal a wealth of fundamental information about listed companies.
- Social Media and News Aggregators: Platforms like Twitter might seem trivial, but they can provide immediate sentiment analysis. Similarly, websites like Reddit have communities dedicated to stock trading, offering a plethora of opinions and insights.
- Economic Reports and Statistics: The Bureau of Economic Analysis publishes crucial economic data which is often used for modeling and analysis in financial markets.
"In trading, you are either ahead of the curve or behind it. Data keeps you informed, prepared for what’s next."
Ultimately, the key for traders lies not just in acquiring vast amounts of data but in selecting the right type of data and learning to synthesize it effectively for their strategies.
Key AI Technologies Shaping Trading
The landscape of stock trading has undergone a major transformation, primarily driven by the integration of Artificial Intelligence technologies. These technologies are not merely add-ons but central components that reshape how trading strategies are devised and executed. Traders today must grasp the significance of these components, as they offer unique benefits and considerations for anyone involved in the stock market—be it a novice or a seasoned expert.
Here, we will explore three major AI technologies that are reshaping stock trading: Machine Learning Algorithms, Natural Language Processing, and Predictive Analytics.
Machine Learning Algorithms
Machine learning stands as a powerhouse in the realm of AI technologies. The core idea revolves around teaching computers how to learn from data patterns without explicitly programmed instructions. This learning ability allows machines to refine their strategies based on historical data, enhancing their effectiveness over time.
- Speed and Accuracy: In the fast-paced world of stock trading, the ability to process vast amounts of data rapidly and accurately is invaluable. Algorithms like neural networks can analyze trends, gauge stock volatility, and predict price movements much faster than humans can.
- Portfolio Management: Machine learning also facilitates the diversification of assets, optimizing portfolios by selecting the most promising stocks while minimizing risk.
- Automated Trading Systems: Companies leverage these algorithms to automate trades. This means when a specific market condition is met, a trade occurs without human intervention, both saving time and reducing emotional trading errors.
To illustrate, consider a hedge fund that uses a machine learning model to analyze patterns from years of stock price data. By identifying past occurrences leading to price surges or drops, the model predicts future movements. This approach streamlines decision-making processes, epitomizing the precision attained through technological integration.
Natural Language Processing in Finance
Natural Language Processing (NLP) is another significant AI technology making waves in stock trading. At its core, NLP focuses on allowing computers to understand, interpret, and generate human language. Its role in finance particularly hinges on analyzing news articles, investor sentiments, and financial reports, translating qualitative data into quantitative insights.
- Sentiment Analysis: By sifting through social media or news sources, NLP can gauge overall market sentiment on particular stocks, providing traders with a pulse on public perception. For instance, major news about a corporation can sway stock prices, and timely insights are critical in such scenarios.
- Data Mining: Investors harness NLP to mine essential insights from extensive text data. This methodology empowers them to make informed decisions based on market commentary, CEO interviews, or even analyst reports.
- Risk Assessment: Advanced NLP techniques can detect potential risks or anomalies in financial documents. Being alerted to emerging issues allows traders to react proactively rather than reactively.
Imagine an investor who uses NLP to analyze a recent market report that reflects a downturn in consumer confidence. By interpreting the nuances of the text, they can initiate timely sell-offs or investments, illustrating how responsive strategies can be shaped through the deft application of language processing.
Predictive Analytics and Its Applications
Predictive analytics is the icing on the cake when it comes to AI technologies in stock trading. These techniques enable traders to look at past data trends and predict potential future outcomes, helping them make educated guesses about market behavior.
- Data-Driven Decision Making: Predictive analytics leads to well-informed decisions backed by concrete evidence rather than gut feeling. By examining past stock performance data, traders can assess risk/return ratios better, ensuring effective strategies.
- Market Simulation: This technology allows traders to model various market scenarios, anticipating how changes in one domain may lead to varied outcomes across the board. Such forecasts can be pivotal during significant economic shifts.
- Customizable Predictions: Different traders may focus on different parameters—be it interest rates, political dynamics, or corporate actions. Predictive analytics can be tailored to individual trading styles, enhancing personalized approaches.
"Data-driven decision making is proving to be the backbone of financial market strategies, where predictive power reshapes risk assessments," notes a recent article on predictive technologies.
In summary, with technologies like machine learning algorithms, natural language processing, and predictive analytics at their disposal, traders are better equipped than ever to navigate an increasingly complex market. Combining these tools not only shapes the efficiency of trading systems but also fosters a more profound understanding of market dynamics, indicating a bright future for AI-assisted trading initiatives.
Advantages of AI in Stock Trading
The world of stock trading has seen unprecedented changes with the rise of artificial intelligence. The integration of AI brings not just a fresh breeze but a whole storm of advantages that many traders, big or small, are learning to leverage. With the ever-moving nature of markets, AI helps in making savvy decisions, streamlining operations, and tightening the reins on risk management, all of which shapes a more efficient trading environment.
Enhanced Decision-Making Capabilities
When it comes to making decisions in the stock market, timing and analysis are everything. AI provides traders with enhanced decision-making capabilities by employing machine learning algorithms that analyze vast datasets at lightning speed. Unlike the average human trader, AI can sift through historical price data, news articles, and social media sentiment simultaneously. For example, suppose an AI program is monitoring trends related to renewable energy stocks. In that case, it can quickly digest data on market swings, regulatory changes, or even consumer sentiment, leading to timely and informed decisions that human traders might miss in the noise.
"The smart use of data creates opportunities that would otherwise remain undiscovered, elevating trading strategies to a whole new level."
Additionally, these systems adapt based on past outcomes, making them not just data-hungry but evolving. With these capabilities, traders can act with a clearer view and often come out ahead of their competitors.
Increasing Efficiency in Trading Operations
Efficiency in trading operations is paramount. AI algorithms process trades and market conditions around the clock without the fatigue humans might face. Think of it: instead of relying solely on a stockbroker's insights during their 9 to 5, AI operates day and night, continuously analyzing market data and executing trades based on pre-defined parameters. This around-the-clock functioning means that AI can capitalize on opportunities that might appear in nanoseconds, thus significantly improving potential profitability.
Moreover, AI enhances efficiency by automating repetitive tasks, such as tracking portfolio performance, managing trades, or executing orders. This means that human traders can focus on developing strategies or engaging with clients rather than getting lost in a sea of spreadsheets and numbers. In the long run, increased efficiency can lead to reduced operational costs and higher profit margins.
Risk Management Done Right
Managing risk is a crucial part of trading that's often overlooked. AI introduces sophisticated risk management techniques that better predict and mitigate potential losses. For instance, traders using AI-powered tools can set customized risk parameters that adjust automatically based on changing market conditions. AI can analyze past market behaviors to gauge risk levels associated with certain trades, providing a proactive stance rather than a reactive one.
Furthermore, AI can simulate various market scenarios and their potential impacts on portfolios, allowing traders to prepare for worst-case situations.
- Assessing historical performance
- Monitoring real-time fluctuations
- Predicting future volatilities
This predictive capability is invaluable, especially in tumultuous market phases where quick decisions can lead to substantial losses. With AI, the approach to risk can shift from merely avoiding pitfalls to strategically navigating through them.
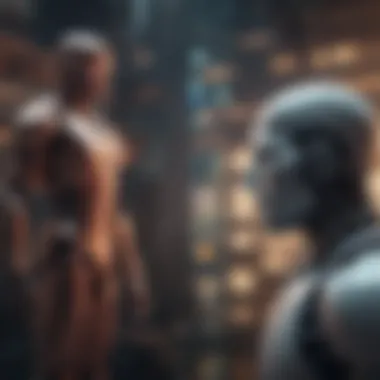
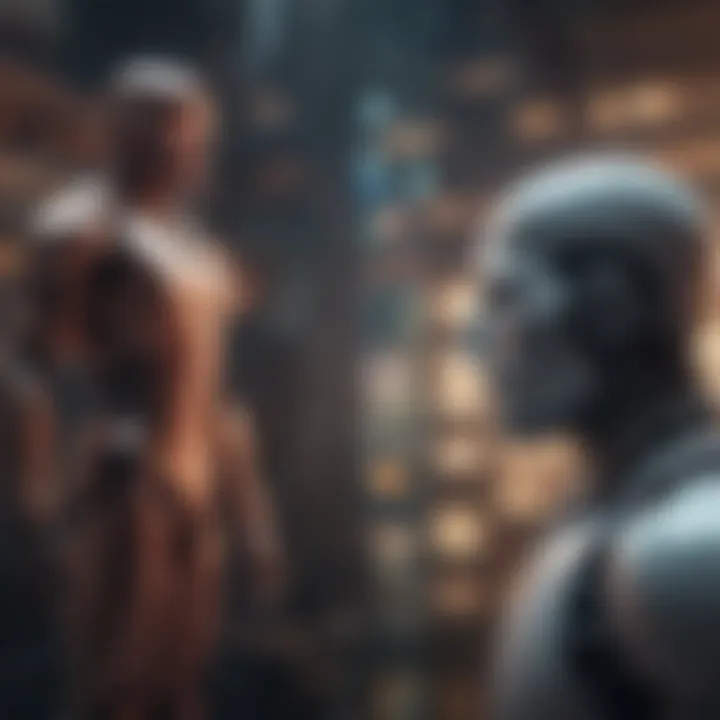
Challenges and Limitations of AI Trading
As we dive into the world of AI stock trading, it’d be foolish to ignore the hurdles that come along. While this technology holds immense promise, it also has its share of challenges, which can significantly shape its effectiveness and reliability. Understanding these complexities can help traders and investors navigate the landscape more judiciously. Whether it’s the quality of data feeding these systems or the unpredictable nature of financial markets, recognizing these limitations is crucial for anyone engaging in AI trading.
Data Quality and Availability Issues
Data is the backbone of any AI system, especially in trading. If the data isn’t up to scratch, the outcomes can be as disappointing as a soggy sandwich. There are two primary concerns here: quality and availability.
First off, low-quality data can skew results, leading to misguided strategies. In finance, where even subtle shifts can make or break a trade, relying on inaccurate information is like trying to find your way with a broken compass. Issues like data inaccuracies, inconsistencies, and incompleteness can arise from various sources, including incorrect market feeds or outdated information. To compound the problem, many data sets could be costly and hard to access, limiting smaller traders and firms.
- Inconsistencies: Different sources may report the same data in various formats, making it hard to create a cohesive picture.
- Missing Information: Key indicators or metrics might be overlooked or simply unavailable, impacting decision-making.
Thus, it's crucial for traders to verify and, when necessary, supplement their data sources. Investing time and resources into reliable data can mean the difference between success and failure.
The Problem of Overfitting
When it comes to machine learning models, overfitting can be a lurking danger. Think of it as a student memorizing facts for an exam without understanding the broader context. The student might ace the test, but when it comes time to apply that knowledge in the real world, they’re lost. In trading, a model that’s too finely tuned to historical data might perform splendidly in backtesting yet falls flat when facing live market conditions.
Overfitting occurs when a model captures noise rather than the underlying trends. This makes it ineffective at predicting future events. How can traders avoid this pitfall? Here are a few strategies:
- Cross-validation: Split data into sets for training and testing to check model accuracy in various conditions.
- Regularization techniques: Reduce the complexity of the model to prevent it from adapting too closely to past data, allowing it to remain more flexible.
Recognizing the signs of overfitting can be the key to maintaining a robust trading strategy that stands the test of time.
Market Volatility and AI Limitations
When it rains, it pours—especially in the world of stock trading. Market volatility can turn the calmest waters into a roaring storm, and AI systems aren’t immune to these fluctuations. One of the cardinal rules to remember is that AI works best under certain conditions. In periods of high volatility, the algorithms can struggle to react swiftly and accurately.
Factors contributing to this challenge include:
- Rapid Market Changes: Sudden news or shifts in economic indicators can lead to erratic price swings, which algorithms may not adapt to quickly enough.
- Black Swan Events: These unforeseen occurrences can completely derail even the most advanced AI models, as they often rely on historical data that doesn't account for such anomalies.
Despite these limitations, it’s important not to discount the potential of AI altogether. Intelligent systems can still provide valuable insights and help traders make informed decisions, as long as they’re with their feet firmly planted on the ground.
"A fool with a tool is still a fool." – This saying perfectly encapsulates the importance of not just relying on AI but supplementing it with human insight and discretion.
Understanding the challenges and limitations of AI in trading arms individuals and firms with the necessary knowledge to mitigate risks and capitalize on opportunities amidst uncertainty. By maintaining an awareness of these factors, traders can harness the power of AI while recognizing its boundaries.
The Impact of AI on Stock Market Dynamics
Artificial Intelligence is reshaping the landscape of stock trading in ways that go beyond individual transactions. Its effects ripple through all aspects of the market, leading to both improvements and new challenges. Understanding these impacts is crucial for any stakeholder in financial markets, from retail investors to seasoned analysts.
Market Efficiency Improvements
AI's ability to analyze vast amounts of data at staggering speeds is one of the primary factors contributing to market efficiency. With algorithms that can process information such as stock prices, trading volumes, and even social media sentiment, AI increases the speed at which trading opportunities are identified. This leads to a more efficient market where prices reflect information more accurately and more quickly.
For instance, machine learning models can sift through news articles, earnings reports, and economic indicators to make predictions about stock movements that a human could take weeks to analyze. As a result, AI enables a reaction time that can capitalize on fleeting market opportunities. The continuous adjustment to new data helps maintain a balance in supply and demand, further stabilizing the markets.
"The potential of AI in stock trading isn't just about speed; it's fundamentally changing the way we interpret market signals and make decisions."
Influences on Traditional Trading Approaches
As AI makes its mark, traditional trading methods are being influenced in profound ways. Traders who relied on rule-based strategies or intuition alone are beginning to incorporate AI insights. This shift can be seen in various forms—from institutional investors employing AI-driven portfolio management tools to retail traders utilizing algorithmic insights delivered through their trading platforms.
In this evolving scenario:
- Algorithmic Trading: Many hedge funds have embraced algorithmic trading, leveraging advanced algorithms to react to market movements almost instantaneously, thereby adapting their strategies based on real-time data inputs.
- Behavioral Adjustments: Traditional traders often rely on set patterns or past experiences. The introduction of AI encourages adaptive trading behaviors, as traders begin to trust AI data and reports.
- Integration of Hybrid Models: There's a rise in hybrid models where human intuition meets AI analytics. Professionals start using AI suggestions as a guiding light rather than absolute directives, blending technological advances with personal insights.
As AI continues to develop, it challenges traders to rethink their approaches for better risk management and opportunities. The market dynamics shift significantly as players adapt to a landscape where AI is a prevailing force, and understanding its impact is essential for staying ahead.
AI Trading Platforms and Tools
Artificial intelligence in stock trading has sparked considerable interest among investors, traders, and market analysts. The advent of AI trading platforms offers a new way to analyze vast datasets, make predictions, and execute trades with precision. These platforms harness the power of machine learning, data analytics, and algorithmic trading methodologies. Understanding which platforms stand out in this rapidly evolving landscape can help traders leverage their potential effectively.
Overview of Leading AI Platforms
There are a bunch of AI trading platforms on the market today, each bringing its own set of features and benefits to the table. Some of the most notable include:
- Tradestation: This platform integrates AI with high-powered analytics, allowing users to develop trading strategies based on historical market data.
- MetaTrader 5: Often used by forex traders, this platform offers robust AI tools for automated trading and analysis, making it a go-to for many.
- Wealthfront: Tailored for everyday investors, Wealthfront provides personalized portfolio management using AI algorithms to automatically adjust your investments based on market conditions.
- Alpaca: With its commission-free trading model, Alpaca enables developers to create their own trading bots. The platform’s API allows for custom algorithmic strategies.
When selecting an AI trading platform, traders should consider factors like ease of use, integration capabilities, and the range of features available. A platform that meets specific trading styles and needs can significantly enhance the trading experience.
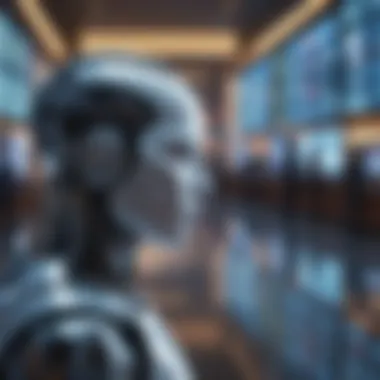
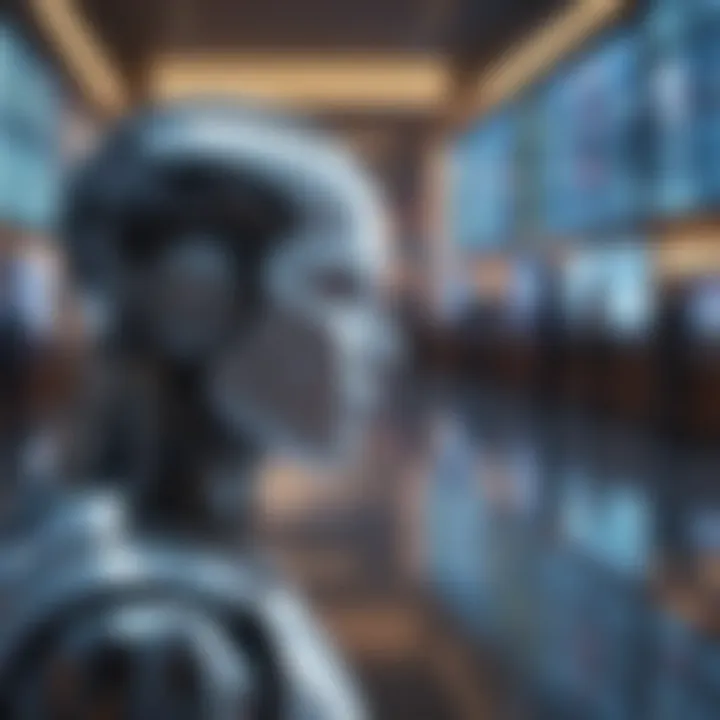
User Experiences and Feedback
Feedback from users of AI trading platforms provides important insights into their effectiveness. Generally, traders appreciate the automation capabilities and the data-driven insights offered.
- Many have noted that these platforms can identify profitable trading opportunities faster than a human trader might. With AI's ability to analyze massive amounts of data, even subtle market patterns can be detected and acted on in a timely manner.
- However, on the flip side, some users caution against over-reliance on AI systems. There are traders who argue that these platforms can miss the nuances of market sentiment that a seasoned trader might catch, leading to missed opportunities or losses in volatile markets.
User feedback indicates a mixed bag: while many traders appreciate the efficiency and informed decision-making AI platforms provide, others express the need for a balanced approach, mixing human intuition with AI's analytical prowess.
"Using AI trading platforms feels like having a personal trading assistant that works around the clock. But I still keep my eye on the ball myself, just in case!"
Real-World Examples of AI in Stock Trading
The use of artificial intelligence in stock trading is increasingly becoming a topic of discussion not only among savvy traders, but also among investment firms looking to gain an edge in the market. Understanding real-world implementations of AI gives traders and analysts clear insights about the effectiveness of these strategies. Real-world examples serve multiple purposes: they not only illustrate successes but also reveal pitfalls to avoid.
Case Studies of Successful AI Implementations
One outstanding example of successful AI integration is AlphaGo, developed by DeepMind. While initially designed for the board game Go, the principles of its neural networks and advanced algorithms paved the way for their application in stock trading. For instance, firms like BlackRock have adopted AI-driven technologies to analyze vast datasets quickly and develop insightful trading strategies. Their Aladdin platform is not just about executing trades; it synthesizes macroeconomic data, individual stock performance, and global market dynamics to offer traders a more complete view of the market landscape. In the end, AI-assisted decision-making led to a marked increase in the firm’s trading efficiency and profitability.
Furthermore, companies like Bridgewater Associates are leveraging AI to refine their investment philosophies and realign portfolio states dynamically. By utilizing AI algorithms to process economic signals more accurately, they can adjust trades almost in real-time, ultimately leading to improved performance in various market conditions.
"AI in trading isn't just a wild card, it’s becoming the playbook that’s reshaping financial strategies."
Lessons Learned from Failures
Despite the tremendous potential, not all AI trading implementations have struck gold. A glaring example is Knight Capital Group, which faced a disastrous malfunction in its trading algorithms in 2012. An error in their AI system resulted in erroneous trades that caused a $440 million loss in under 30 minutes. This failure highlighted the crucial importance of testing and validating AI systems rigorously before deploying them in live trading environments.
Moreover, firms external to the finance sector that attempted to apply AI models from other industries without proper adjustment often hit roadblocks. For instance, a tech startup that used AI algorithms primarily designed for retail forecasting tried to adapt them for stock trading without recognizing the fundamentally different variables at play. The result was inconsistent trading outcomes where numerous trades were executed, but many positions floundered when exposed to market volatility.
Overall, these real-world examples lay out valuable insights. Successful AI implementations can drive efficiency and improve decision-making, but without due diligence and suitable model adaptation, the pitfalls can lead to losses that would make even the savviest traders shiver.
Future Trends in AI Stock Trading
Understanding the trends shaping AI in stock trading is essential for anyone looking to stay ahead of the curve. These trends promise not just to influence the landscape of trading but to redefine how assets are evaluated, managed, and optimized. If you’ve been keeping an ear to the ground, the buzz around AI technologies is hard to ignore. One aspect that stands out is how AI adoption is no longer limited to large institutional investors; rather, it’s spreading like wildfire across retail investors as well.
The Role of AI in Algorithmic Trading
Algorithmic trading refers to the use of AI-driven algorithms to execute trades at speeds and frequencies far beyond human capabilities. This method enables traders to capitalize on many market signals quickly. Nowadays, these algorithms can adjust strategies based on real-time data, pulling from myriad information sources, be it social media sentiment or economic indicators.
The adaptability in algorithmic strategies plays a crucial part in today's dynamic markets. For instance, consider a trading algorithm designed to operate only during high-volume trading hours. Thanks to machine learning, these algorithms can learn from past data, ensuring they improve continuously. It’s like having a seasoned veteran behind the scenes, making quick but informed decisions.
"With AI, traders can adapt their strategies in real-time, tapping into patterns that human traders might miss."
Moreover, many users find AI trading platforms intuitive and user-friendly, lowering the barrier to entry for those new to investment strategies. This development in algorithmic trading not only democratizes access to sophisticated trading strategies but fosters a greater understanding of market mechanisms among all traders.
Emerging Technologies on the Horizon
As one peers into the future, several technologies are on the brink of significant impact within AI-driven trading environments. One technology to watch is quantum computing, which provides unprecedented processing power. If successfully integrated, it can enhance algorithm efficiency and potentially uncover hidden patterns in stock market data that classical computers simply can’t handle in a reasonable timeframe.
Additionally, blockchain technology is promising for improving transaction transparency and security. Its potential applications range from enhancing the reliability of trading data to lowering cost structures associated with conventional trading methods. As blockchain gains traction, expect a surge in decentralized finance (DeFi) platforms. These platforms may alter how traditional stock trading is done, particularly concerning intermediation and settlement processes.
On another front, natural language processing is set to evolve. Current models are getting more sophisticated, enabling them to analyze news, earnings calls, and social media platforms. The implications of this are massive; traders will gain insights that were previously lost in vast amounts of unstructured data, making it easier to predict market trends.
The future is brimming with potential, and as technologies mature, they will likely engender more profound changes in trading practices than previously thought possible.
Culmination
In closing, the incorporation of artificial intelligence in stock trading signifies not just a trend, but a transformative shift in how investors approach the market. The insights gleaned from this exploration underscore the resourcefulness of AI as a tool that goes beyond the capabilities of traditional trading methods.
Summarizing Key Insights
One of the primary takeaways from the article is the sheer volume of data AI can process. Unlike a human trader, who may sift through various reports and news articles, AI can analyze vast datasets at remarkable speed. This ability enables traders to uncover patterns that might otherwise remain hidden, providing a significant edge in making informed decisions.
Moreover, the evolving nature of trading strategies demonstrates AI's adaptability in a constantly shifting financial landscape. It's clear that traders leveraging these technologies are better positioned to identify opportunities and mitigate risks compared to those relying solely on instinct or traditional indicators.
- Enhanced Decision-Making: AI improves the decision-making process, empowering traders to act on comprehensive data insights rather than subjective interpretations.
- Efficient Operations: The automation of trading processes not only reduces operational costs but also increases the speed at which trades can be executed.
- Robust Risk Management: AI tools can model complex scenarios, enabling traders to prepare for market fluctuations and other uncertainties with greater confidence.
The Way Forward for Traders
Looking ahead, traders must embrace the reality that AI is reshaping the financial markets. Understanding how to integrate these advanced tools into their strategies is essential. Closet tech enthusiasts can benefit from becoming well-versed in the various AI platforms available today, such as Alpaca or QuantConnect, exploring their unique offerings tied to stock trading.
Additionally, there's a clear demand for continuous learning in this field. Engaging with communities, whether on platforms like Reddit or Facebook, or keeping up-to-date with articles on sources like Britannica or Wikipedia, can provide invaluable knowledge and fresh perspectives.
Investors who resist this change may find themselves left behind as those who adopt new technologies continue to gain market share. Thus, it's prudent for all stock traders to remain open to the ongoing advancements in AI, tailoring their approaches to weave in these innovative solutions, resulting in a more thorough understanding of the market dynamics.
"In an era where technology dictates pace, adaptability is the key to success in trading."
In summary, staying informed and adaptable can aid traders in not just surviving, but thriving in this evolving landscape driven by artificial intelligence.